Articles by tag: Verification
User Studies of Principled Model Finder Output
Tierless Programming for SDNs: Differential Analysis
Tierless Programming for SDNs: Verification
Tierless Programming for SDNs: Optimality
Tierless Programming for SDNs: Events
Tierless Programming for Software-Defined Networks
Verifying Extensions’ Compliance with Firefox's Private Browsing Mode
Aluminum: Principled Scenario Exploration Through Minimality
ADsafety
Crowdsourcing User Studies for Formal Methods
Tags: Verification, User Studies
Posted on 03 July 2017.For decades, we have neglected performing serious user studies of formal-methods tools. This is now starting to change. An earlier post introduces our new work in this area.
That study works with students in an upper-level class, who are a fairly good proxy for some developers (and are anyway an audience we have good access to). Unfortunately, student populations are problematic for several reasons:
-
There are only so many students in a class. There may not be enough to obtain statistical strength, especially on designs that require A/B testing and the like.
-
The class is offered only so often. It may take a whole year between studies. (This is a common problem in computing education research.)
-
As students progress through a class, it’s hard to “rewind” them and study their responses at an earlier stage in their learning.
And so on. It would be helpful if we could obtain large numbers of users quickly, relatively cheaply, and repeatedly.
This naturally suggests crowdsourcing. Unfortunately, the tasks we are examining involve using tools based on formal logic, not identifying birds or picking Web site colors (or solving CAPTCHAs…). That would seem to greatly limit the utility of crowd-workers on popular sites like Mechanical Turk.
In reality, this depends on how the problem is phrased. If we view it as “Can we find lots of Turkers with knowledge of Promela (or Alloy or …)?”, the answer is pretty negative. If, however, we can rework the problems somewhat so the question is “Can we get people to work on a puzzle?”, we can find many, many more workers. That is, sometimes the problem is one of vocabulary (and in particular, the use of specific formal methods languages) than of raw ability.
Concretely, we have taken the following steps:
-
Adapt problems from being questions about Alloy specifications to being phrased as logic “puzzles”.
-
Provide an initial training phase to make sure workers understand what we’re after.
-
Follow that with an evaluation phase to ensure that they “got the idea”. Only consider responses from those workers who score at a high enough threshold on evaluation.
-
Only then conduct the actual study.
Observe that even if we don’t want to trust the final results obtained from crowdsourcing, there are still uses for this process. Designing a good study requires several rounds of prototyping: even simple wording choices can have huge and unforeseen (negative) consequences. The more rounds we get to test a study, the better it will come out. Therefore, the crowd is useful at least to prototype and refine a study before unleashing it on a more qualified, harder-to-find audience — a group that, almost by definition, you do not want to waste on a first-round study prototype.
For more information, see our paper. We find fairly useful results using workers on Mechanical Turk. In many cases the findings there correspond with those we found with class students.
User Studies of Principled Model Finder Output
Tags: Verification, User Studies
Posted on 01 July 2017.For decades, formal-methods tools have largely been evaluated on their correctness, completeness, and mathematical foundations while side-stepping or hand-waving questions of usability. As a result, tools like model checkers, model finders, and proof assistants can require years of expertise to negotiate, leaving knowledgeable but uninitiated potential users at a loss. This state of affairs must change!
One class of formal tool, model finders, provides concrete instances of a specification, which can guide a user’s intuition or witness the failure of desired properties. But are the examples produced actually helpful? Which examples ought to be shown first? How should they be presented, and what supplementary information can aid comprehension? Indeed, could they even hinder understanding?
We’ve set out to answer these questions via disciplined user-studies. Where can we find participants for these studies? Ideally, we would survey experts. Unfortunately, it has been challenging to do so in the quantities needed for statistical power. As an alternative, we have begun to use formal methods students in Brown’s upper-level Logic for Systems class. The course begins with Alloy, a popular model-finding tool, so students are well suited to participate in basic studies. With this population, we have found some surprising results that call into question some intuitively appealing answers to (e.g.) the example-selection question.
For more information, see our paper.
Okay, that’s student populations. But there are only so many students in a class, and they take the class only so often, and it’s hard to “rewind” them to an earlier point in a course. Are there audiences we can use that don’t have these problems? Stay tuned for our next post.
Tierless Programming for SDNs: Differential Analysis
Tags: Flowlog, Programming Languages, Software-Defined Networking, Verification
Posted on 02 June 2015.
This post is part of our series about tierless network programming with Flowlog:
Part 1: Tierless Programming
Part 2: Interfacing with External Events
Part 3: Optimality
Part 4: Verification
Part 5: Differential Analysis
Verification is a powerful way to make sure a program meets expectations, but what if those expectations aren't written down, or the user lacks the expertise to write formal properties? Flowlog supports a powerful form of property-free analysis: program differencing.
When we make a program change, usually we're starting from a version that "works". We'd like to transfer what confidence we had in the original version to the new version, plus confirm our intuition about the changes. In other words, even if the original program had bugs, we'd like to at least confirm that the edit doesn't introduce any new ones.
Of course, taking the syntactic difference of two programs is easy — just use diff! — but usually that's not good enough. What we want is the behavioral, or semantic difference. Flowlog provides semantic differencing via Alloy, similarly to how it does property checking. We call Flowlog's differencing engine Chimp (short for Change-impact).
Differences in Output and State Transitions
Chimp translates both the old (prog1) and new (prog2) versions to Alloy, then supports asking questions like: Will the two versions ever handle packets differently? More generally, we can ask Chimp whether the program's output behavior ever differs: does there exist some program state and input event such that, in that state, the two programs will disagree on output?
pred changePolicyOut[st: State, ev: Event] { some out: Event | prog1/outpolicy[st,ev,out] && not prog2/outpolicy[st,ev,out] || prog2/outpolicy[st,ev,out] && not prog1/outpolicy[st,ev,out] }Any time one program issues an output event that the other doesn't, Chimp displays an Alloy scenario.
We might also ask: When can the programs change state differently? Similarly to changePolicyOut above, Chimp defines changeStateTransition[st: State, ev: Event] as matching any of the following for each table T in the program:
some x0, ..., xn: univ | prog1/afterEvent_T[prestate, ev, x0, ..., xn] && not prog2/afterEvent_T[prestate, ev, x0, ..., xn] || prog2/afterEvent_T[prestate, ev, x0, ..., xn] && not prog1/afterEvent_T[prestate, ev, x0, ..., xn]
Recall that afterEvent_T keeps track of when each tuple is in the table T after an event is processed.
Refining Differential Analysis
The two predicates above are both built into Chimp. Using them as a starting point, users can ask pointed questions about the effects of the change. For instance, will any TCP packets be handled differently? Just search for a pre-state and a TCP packet that the programs disagree on:
some prestate: State, p: EVtcp_acket | changePolicyOut[prestate, p]This lets users explore the consequences of their change without any formal guidance except their intuition about what the change should do.
Reachability
So far, these queries show scenarios where the programs differ, taking into consideration all potential inputs and starting states; this includes potentially unreachable starting states. We could, for instance, have two programs that behave differently if a table is populated (resulting in a non-empty semantic diff!) yet never actually insert rows into that table. Chimp provides optional reachability-checking to counter this, although users must cap the length of system traces being searched.
Schema Clashes
Suppose that we want to modify the original source-tracking example to keep track of flows by source and destination, rather than just source addresses. Now instead of one column:
TABLE seen(macaddr);the seen table has two columns:
TABLE seen(macaddr, macaddr);
This poses a challenge for Chimp; what shape should the seen table be? If Chimp finds a scenario, should it show a seen table with one or two columns? We call this situation a schema clash, and Chimp addresses it by creating two separate tables in the prestate: one with one column (used by the first program) and another with two columns (used by the second program).
Doing this causes a new issue: Chimp searches for arbitrary states that satisfy the change-impact predicates. Since there is no constraint between the values of the two tables, Chimp might return a scenario where (say) the first seen table is empty, but the second contains tuples!
This doesn't match our intuition for the change: we expect that for every source in the first table, there is a source-destination pair in the second table, and vice versa. We can add this constraint to Chimp and filter the scenarios it shows us, but first, we should ask whether that constraint actually reflects the behavior of the two programs.
Differential Properties
Since it's based on Flowlog's verification framework, Chimp allows us to check properties stated over multiple programs. Our expecation above, stated in Alloy for an arbitrary state s, is:
all src: Macaddr | src in s.seen1 iff some dst: Macaddr | src->dst in s.seen2
Let's check that this condition holds for all reachable states. We'll proceed inductively. The condition holds trivially at the starting (empty) state; so we only need to show that it is preserved as the program transitions. We search for a counterexample:
some prestate: State, ev: Event | { // prestate satisfies the condition all src: Macaddr | src in prestate.seen_1 iff some dst: Macaddr | src->dst in prestate.seen_2 // poststate does not some src: Macaddr | (prog1/afterEvent_seen_1[prestate,ev,src] and all dst: Macaddr | not prog2/afterEvent_seen_2[prestate,ev,src,dst]) || (not prog1/afterEvent_seen_1[prestate,ev,src] and some dst: Macaddr | prog2/afterEvent_seen_2[prestate,ev,src,dst]) }
Chimp finds no counterexample. Unfortunately, Chimp can't guarantee that this isn't a false negative; the query falls outside the class where Chimp can guarantee a complete search. Nevertheless, the lack of counterexample serves to increase our confidence that the change respects our intent.
After adding the constraint that, for every source in the first table, there is a source-destination pair in the second table, Chimp shows us that the new program will change the state (to add a new destination) even if the source is already in seen.
Further Reading
Chimp supports more features than discussed here; for instance, Chimp can compare the behavior of any number of program versions, but a two-program comparison is the most common. You can read more about Chimp in our paper.
Tierless Programming for SDNs: Verification
Tags: Flowlog, Programming Languages, Software-Defined Networking, Verification
Posted on 17 April 2015.
This post is part of our series about tierless network programming with Flowlog:
Part 1: Tierless Programming
Part 2: Interfacing with External Events
Part 3: Optimality
Part 4: Verification
Part 5: Differential Analysis
The last post said what it means for Flowlog's compiler to be optimal, which prevents certain bugs from ever occurring. But what about the program itself? Flowlog has built-in features to help verify program correctness, independent of how the network is set up.
To see Flowlog's program analysis in action, let's first expand our watchlist program a bit more. Before, we just flooded packets for demo purposes:
DO forward(new) WHERE new.locPt != p.locPt;Now we'll do something a bit smarter. We'll make the program learn which ports lead to which hosts, and use that knowledge to avoid flooding when possible (this is often called a "learning switch"):
TABLE learned(switchid, portid, macaddr); ON packet(pkt): INSERT (pkt.locSw, pkt.locPt, pkt.dlSrc) INTO learned; DO forward(new) WHERE learned(pkt.locSw, new.locPt, pkt.dlDst); OR (NOT learned(pkt.locSw, ANY, pkt.dlDst) AND pkt.locPt != new.locPt);The learned table stores knowledge about where addresses have been seen on the network. If a packet arrives with a destination the switch has seen before as a source, there's no need to flood! While this program is still fairly naive (it will fail if the network has cycles in it) it's complex enough to have a few interesting properties we'd like to check. For instance, if the learned table ever holds multiple ports for the same switch and address, the program will end up sending multiple copies of the same packet. But can the program ever end up in such a state? Since the initial, startup state is empty, this amounts to checking:
Verifying Flowlog
Each Flowlog rule defines part of an event-handling function saying how the system should react to each packet seen. Rules compile to logical implications that Flowlog's runtime interprets whenever a packet arrives.
Alloy is a tool for analyzing relational logic specifications. Since Flowlog rules compile to logic, it's easy to describe in Alloy how Flowlog programs behave. In fact, Flowlog can automatically generate Alloy specifications that describe when and how the program takes actions or changes its state.For example, omitting some Alloy-language foibles for clarity, here's how Flowlog describes our program's forwarding behavior in Alloy.
pred forward[st: State, p: EVpacket, new: EVpacket] { // Case 1: known destination (p.locSw->new.locPt->p.dlDst) in learned and (p.locSw->new.locPt) in switchHasPort and ...) or // Case 2: unknown destination (all apt: Portid | (p.locSw->apt->p.dlDst) not in learned and new.locPt != p.locPt and (p.locSw->new.locPt) in switchHasPort and ...) }An Alloy predicate is either true or false for a given input. This one says whether, in a given state st, an arbitrary packet p will be forwarded as a new packet new (containing the output port and any header modifications). It combines both forwarding rules together to construct a logical definition of forwarding behavior, rather than just a one-way implication (as in the case of individual rules).
The automatically generated specification also contains other predicates that say how and when the controller's state will change. For instance, afterEvent_learned, which says when a given entry will be present in learned after the controller processes a packet. An afterEvent predicate is automatically defined for every state table in the program.
Using afterEvent_Learned, we can verify our goal: that whenever an event ev arrives, the program will never add a second entry (sw, pt2,addr) to learned:
assert FunctionalLearned { all pre: State, ev: Event | all sw: Switchid, addr: Macaddr, pt1, pt2: Portid | (not (sw->pt1->addr in pre.learned) or not (sw->pt2->addr in pre.learned)) and afterEvent_learned[pre, ev, sw, pt1, addr] and afterEvent_learned[pre, ev, sw, pt2, addr] implies pt1 = pt2 }
Alloy finds a counterexample scenario (in under a second):
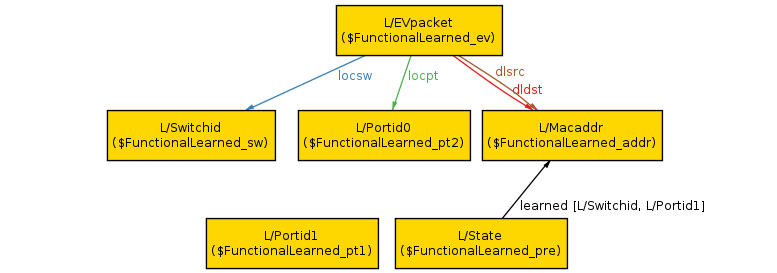
The scenario shows an arbitrary packet (L/EVpacket; the L/ prefix can be ignored) arriving at port 1 (L/Portid1) on an arbitrary switch (L/Switchid). The packet has the same source and destination MAC address (L/Macaddr). Before the packet arrived, the controller state had a single row in its learned table; it had previously learned that L/Macaddr can be reached out port 0 (L/Portid1). Since the packet is from the same address, but a different port, it will cause the controller to add a second row to its learned table, violating our property.
This situation isn't unusual if hosts are mobile, like laptops on a campus network are. To fix this issue, we add a rule that removes obsolete mappings from the table:
DELETE (pkt.locSw, pt, pkt.dlSrc) FROM learned WHERE not pt = pkt.locPt;Alloy confirms that the property holds of the modified program. We now know that any reachable state of our program is valid.
Verification Completeness
Alloy does bounded verification: along with properties to check, we provide concrete bounds for each datatype. We might say to check up to to 3 switches, 4 IP addresses, and so on. So although Alloy never returns a false positive, it can in general produce false negatives, because it searches for counterexamples only up to the given bounds. Fortunately, for many useful properties, we can compute and assert a sufficient bound. In the property we checked above, a counterexample needs only 1 State (to represent the program's pre-state) and 1 Event (the arriving packet), plus room for its contents (2 Macaddrs for source and destination, etc.), along with 1 Switchid, 2 Portids and 1 Macaddr to cover the possibly-conflicted entries in the state. So when Alloy says that the new program satisfies our property, we can trust it.
Benefits of Tierlessness
Suppose we enhanced the POX version of this program (Part 1) to learn ports in the same way, and then wanted to check the same property. Since the POX program explicitly manages flow-table rules, and the property involves a mixture of packet-handling (what is sent up to the controller?) and controller logic (how is the state updated?), checking the POX program would mean accounting for those rules and how the controller updates them over time. This isn't necessary for the Flowlog version, because rule updates are all handled optimally by the runtime. This means that property checking is simpler: there's no multi-tiered model of rule updates, just a model of the program's behavior.
You can read more about Flowlog's analysis support in our paper.
In the next post, we'll finish up this sequence on Flowlog by reasoning about behavioral differences between multiple versions of the same program.
Tierless Programming for SDNs: Optimality
Tags: Flowlog, Programming Languages, Software-Defined Networking, Verification
Posted on 13 April 2015.
This post is part of our series about tierless network programming with Flowlog:
Part 1: Tierless Programming
Part 2: Interfacing with External Events
Part 3: Optimality
Part 4: Verification
Part 5: Differential Analysis
Since packets can trigger controller-state updates and event output, you might wonder exactly which packets a Flowlog controller needs to see. For instance, a packet without a source in the watchlist will never alter the controller's state. Does such a packet need to grace the controller at all? The answer is no. In fact, there are only three conditions under which switch rules do not suffice, and the controller must be involved in packet-handling:
- when the packet will cause a change in controller state;
- when the packet will cause the controller to send an event; and
- when the packet must be modified in ways that OpenFlow 1.0 does not support on switches.
Flowlog's compiler ensures the controller sees packets if and only if one of these holds; the compiler is therefore optimal with respect to this list. To achieve this, the compiler analyzes every packet-triggered statement in the program. For instance, the INSERT statement above will only change the state for packets with a source in the watchlist (a condition made explicit in the WHERE clause) and without a source in the seen table (implicit in Flowlog's logical semantics for INSERT). Only if both of these conditions are met will the controller see a packet. An optimal compiler prevents certain kinds of bugs from occurring: the controller program will never miss packets that will affect its state, and it will never receive packets it doesn't need.
You can read more about Flowlog in our paper.
In the next post, we'll look at Flowlog's built-in verification support.
Tierless Programming for SDNs: Events
Tags: Flowlog, Programming Languages, Software-Defined Networking, Verification
Posted on 01 March 2015.
This post is part of our series about tierless network programming with Flowlog:
Part 1: Tierless Programming
Part 2: Interfacing with External Events
Part 3: Optimality
Part 4: Verification
Part 5: Differential Analysis
The last post introduced Flowlog, a tierless language for SDN controller programming. You might be wondering, "What can I write in Flowlog? How expressive is it?" To support both its proactive compiler and automated program analysis (more on this in the next post) we deliberately limited Flowlog's expressive power. There are no loops in the language, and no recursion. Instead of trying to be universally expressive, Flowlog embraces the fact that most programs don't run in a vacuum. A controller may need to interact with other services, and developers may wish to re-use pre-existing code. To enable this, Flowlog programs can call out to non-Flowlog libraries. The runtime uses standard RPCs (Thrift) for inter-process communication, so existing programs can be quickly wrapped to communicate with Flowlog. Much like how Flowlog abstracts out switch-rule updates, it also hides the details of inter-process communcation. To see this, let's enhance the address-logger application with a watch-list that external programs can add to. We need a new table ("watchlist"), populated by arriving "watchplease" events that populate the table. Finally, we make sure only watched addresses are logged:
TABLE seen(macaddr); TABLE watchlist(macaddr); EVENT watchplease = {target: macaddr}; ON watchplease(w): INSERT (w.target) INTO watchlist; ON packet(p): INSERT (p.dlSrc) INTO seen WHERE watchlist(p.dlSrc); DO forward(new) WHERE new.locPt != p.locPt;When the program receives a watchplease event (sent via RPC from an external program) it adds the appropriate address to its watchlist.
Sending Events
Flowlog programs can also send events. Suppose we want to notify some other process when a watchlisted address is seen, and the process is listening on TCP port 20000. We just declare a named pipe that carries notifications to that port:EVENT sawaddress = {addr: macaddr}; OUTGOING sendaddress(sawaddress) THEN SEND TO 127.0.0.1:20000;and then write a notification to that pipe for appropriate packets:
ON packet(p) WHERE watchlist(p.dlSrc): DO sendaddress(s) WHERE s.addr = p.dlSrc;
Synchronous Communication
The event system supports asynchronous communication, but Flowlog also allows synchronous queries to external programs. It does this with a remote state abstraction. If we wanted to manage the watchlist remotely, rather than writingTABLE watchlist(macaddr);we would write:
REMOTE TABLE watchlist(macaddr) FROM watchlist AT 127.0.0.1 20000 TIMEOUT 10 seconds;which tells Flowlog it can obtain the current list by sending queries to port 20000. Since these queries are managed behind the scenes, the program doesn't need to change—as far as the programmer is concerned, a table is a table. Finally, the timeout says that Flowlog can cache prior results for 10 seconds.
Interfacing External Programs with Flowlog
Flowlog can interface with code in any language that supports Thrift RPC (including C++, Java, OCaml, and many others). To interact with Flowlog, one only needs to implement the interface Flowlog requires: a function that accepts notifications and a function that responds to queries. Other functions may also (optionally) send notifications. Thrift's library handles the rest.You can read more about Flowlog's events in our paper.
In the next post, we'll look at what it means for Flowlog's compiler to be optimal.
Tierless Programming for Software-Defined Networks
Tags: Flowlog, Programming Languages, Software-Defined Networking, Verification
Posted on 30 September 2014.
This post is part of our series about tierless network programming with Flowlog:
Part 1: Tierless Programming
Part 2: Interfacing with External Events
Part 3: Optimality
Part 4: Verification
Part 5: Differential Analysis
Network devices like switches and routers update their behavior in real-time. For instance, a router may change how it forwards traffic to address an outage or congestion. In a traditional network, devices use distributed protocols to decide on mutually consistent behavior, but Software-Defined Networks (SDN) operate differently. Switches are no longer fully autonomous agents, but instead receive instructions from logically centralized controller applications running on separate hardware. Since these applications can be arbitrary programs, SDN operators gain tremendous flexibility in customizing their network.
The most popular SDN standard in current use is OpenFlow. With OpenFlow, Controller applications install persistent forwarding rules on the switches that match on packet header fields and list actions to take on a match. These actions can include header modifications, forwarding, and even sending packets to the controller for further evaluation. When a packet arrives without a matching rule installed, the switch defaults to sending the packet to the controller for instructions.
Let's write a small controller application. It should (1) record the addresses of machines sending packets on the network and (2) cause each switch to forward traffic by flooding (i.e., sending out on all ports except the arrival port). This is simple enough to write in POX, a controller platform for Python. The core of this program is a function that reacts to packets as they arrive at the controller (we have removed some boilerplate and initialization):
def _handle_PacketIn (self, event): packet = event.parsed def install_nomore (): msg = of.ofp_flow_mod() msg.match = of.ofp_match(dl_src = packet.src) msg.buffer_id = event.ofp.buffer_id msg.actions.append(of.ofp_action_output(port = of.OFPP_FLOOD)) self.connection.send(msg) def do_flood (): msg = of.ofp_packet_out() msg.actions.append(of.ofp_action_output(port = of.OFPP_FLOOD)) msg.data = event.ofp msg.buffer_id = None msg.in_port = event.port self.connection.send(msg) self.seenTable.add(packet.src) install_nomore() do_flood()
First, the controller records the packet's source in its internal table. Next, the install_nomore function adds a rule to the switch saying that packets with this source should be flooded. Once the rule is installed, the switch will not send packets with the same source to the controller again. Finally, the do_flood function sends a reply telling the switch to flood the packet.
This style of programming may remind you of the standard three-tier web-programming architecture. Much like a web program generates JavaScript or SQL strings, controller programs produce new switch rules in real-time. One major difference is that switch rules are much less expressive than JavaScript, which means that less computation can be delegated to the switches. A bug in a controller program can throw the entire network's behavior off. But it's easy to introduce bugs when every program produces switch rules in real-time, effectively requiring its own mini-compiler!
SDN Programming Without Tiers
We've been working on a tierless language for SDN controllers: Flowlog. In Flowlog, you write programs as if the controller sees every packet, and never have to worry about the underlying switch rules. This means that some common bugs in controller/switch interaction can never occur, but it also means that the programming experience is simpler. In Flowlog, our single-switch address-monitoring program is just:
TABLE seen(macaddr); ON ip_packet(p): INSERT (p.dlSrc) INTO seen; DO forward(new) WHERE new.locPt != p.locPt;
The first line declares a one-column database table, "seen". Line 2 says that the following two lines are triggered by IP packets. Line 3 adds those packets' source addresses to the table, and line 4 sends the packets out all other ports.
As soon as this program runs, the Flowlog runtime proactively installs switch rules to match the current controller state and automatically ensures consistency. As the controller sees more addresses, the switch sends fewer packets back to the controller—but this is entirely transparent to the programmer, whose job is simplified by the abstraction of an all-seeing controller.
Examples and Further Reading
Flowlog is good for more than just toy examples. We've used Flowlog for many different network applications: ARP-caching, network address translation, and even mediating discovery and content-streaming for devices like Apple TVs. You can read more about Flowlog and Flowlog applications in our paper.
The next post talks more about what you can use Flowlog to write, and also see how Flowlog allows programs to call out to external libraries in other languages.
Verifying Extensions’ Compliance with Firefox's Private Browsing Mode
Tags: Browsers, JavaScript, Programming Languages, Security, Verification, Types
Posted on 19 August 2013.All modern browsers now support a “private browsing mode”, in which the browser ostensibly leaves behind no traces on the user's file system of the user's browsing session. This is quite subtle: browsers have to handle caches, cookies, preferences, bookmarks, deliberately downloaded files, and more. So browser vendors have invested considerable engineering effort to ensure they have implemented it correctly.
Firefox, however, supports extensions, which allow third party code to run with all the privilege of the browser itself. What happens to the security guarantee of private browsing mode, then?
The current approach
Currently, Mozilla curates the collection of extensions, and any extension must pass through a manual code review to flag potentially privacy-violating behaviors. This is a daunting and tedious task. Firefox contains well over 1,400 APIs, of which about twenty are obviously relevant to private-browsing mode, and another forty or so are less obviously relevant. (It depends heavily on exactly what we mean by the privacy guarantee of “no traces left behind”: surely the browser should not leave files in its cache, but should it let users explicitly download and save a file? What about adding or deleting bookmarks?) And, if the APIs or definition of private-browsing policy ever change, this audit must be redone for each of the thousands of extensions.
The asymmetry in this situation should be obvious: Mozilla auditors should not have to reconstruct how each extension works; it should be the extension developers' responsibility to convince the auditor that their code complies with private-browsing guarantees. After all, they wrote the code! Moreover, since auditors are fallible people, too, we should look to (semi-)automated tools to lower their reviewing effort.
Our approach
So what property, ultimately, do we need to confirm about an extension's code to ensure its compliance? Consider the pseudo-code below, which saves the current preferences to disk every few minutes:
var prefsObj = ...
const thePrefsFile = "...";
function autoSavePreferences() {
if (inPivateBrowsingMode()) {
// ...must store data only in memory...
return;
} else {
// ...allowed to save data to disk...
var file = openFile(thePrefsFile);
file.write(prefsObj.tostring());
}
}
window.setTimeout(autoSafePreferences, 3000);
The key observation is that this code really defines two programs that happen to share the same source code: one program runs when the browser is in private browsing mode, and the other runs when it isn't. And we simply do not care about one of those programs, because extensions can do whatever they'd like when not in private-browsing mode. So all we have to do is “disentangle” the two programs somehow, and confirm that the private-browsing version does not contain any file I/O.
Technical insight
Our tool of choice for this purpose is a type system for JavaScript. We've used such a system before to analyze the security of the ADsafe sandbox. The type system is quite sophisticated to handle JavaScript idioms precisely, but for our purposes here we need only part of its expressive power. We need three pieces: first, three new types; second, specialized typing rules; and third, an appropriate type environment.
- We define one new primitive type:
Unsafe
. We will ascribe this type to all the privacy-relevant APIs. - We use union types to define
Ext
, the type of “all private-browsing-safe extensions”, namely: numbers, strings, booleans, objects whose fields areExt
, and functions whose argument and return types areExt
. Notice thatUnsafe
“doesn’t fit” intoExt
, so attempting to use an unsafe function, or pass it around in extension code, will result in a type error. - Instead of defining
Bool
as a primitive type, we will instead defineTrue
andFalse
as primitive types, and defineBool
as their union.
- If an expression has some union type, and only one component
of that union actually typechecks, then we optimistically say
that the expression typechecks even with the whole union type.
This might seem very strange at first glance: surely, the
expression
5("true")
shouldn't typecheck? But remember, our goal is to prevent privacy violations, and the code above will simply crash---it will never write to disk. Accordingly, we permit this code in our type system. - We add special rules for typechecking if-expressions. When
the condition typechecks at type
True
, we only check the then-branch; when the condition typechecks at typeFalse
, we only check the else-branch. (Otherwise, we check both branches as normal.)
- We give all the privacy-relevant APIs the
type
Unsafe
. - We give the API
inPrivateBrowsingMode()
the typeTrue
. Remember: we just don't care what happens when it's false!
Put together, what do all these pieces achieve?
Because Unsafe
and Ext
are disjoint from
each other, we can safely segregate any code into two pieces that
cannot communicate with each other. By carefully initializing the
type environment, we make Unsafe
precisely delineate
the APIs that extensions should not use in private browsing mode.
The typing rules for if-expressions plus the type
for inPrivateBrowsingMode()
amount to disentangling the
two programs from each other: essentially, it implements dead-code
elimination at type-checking time. Lastly, the rule about union
types makes the system much easier for programmers to use, since they
do not have to spend any effort satisfying the typechecker about
properties other than this privacy guarantee.
In short, if a program passes our typechecker, then it must not call any privacy-violating APIs while in private-browsing mode, and hence is safe. No audit needed!
Wait, what about exceptions to the policy?
Sometimes, extensions have good reasons for writing to disk even
while in private-browsing mode. Perhaps they're updating their
anti-phishing blacklists, or they implement a download-helper that
saves a file the user asked for, or they are a bookmark manager. In
such cases, there simply is no way for the code to typecheck. As in
any type system, we provide a mechanism to break out of the type
system: an unchecked typecast. We currently write such casts
as cheat(T)
. Such casts must be checked by a human
auditor: they are explicitly marking the places where the extension
is doing something unusual that must be confirmed.
(In our original version, we took our cue from Haskell and wrote
such casts as unsafePerformReview
, but sadly that is
tediously long to write.)
But does it work?
Yes.
We manually analyzed a dozen Firefox extensions that had already
passed Mozilla's auditing process. We annotated the extensions with
as few type annotations as possible, with the goal of forcing the
code to pass the typechecker, cheat
ing if necessary.
These annotations found five extensions that violated
the private-browsing policy: they could not be typechecked without
using cheat
, and the unavoidable uses
of cheat
pointed directly to where the extensions
violated the policy.
Further reading
We've written up our full system, with more formal definitions of the types and worked examples of the annotations needed. The writeup also explains how we create the type environment in more detail, and what work is necessary to adapt this system to changes in the APIs or private-browsing implementation.
Aluminum: Principled Scenario Exploration Through Minimality
Tags: Verification
Posted on 13 May 2013.Software artifacts are hard to get right. Not just programs, but protocols, configurations, etc. as well! Analysis tools can help mitigate the risk at every stage in the development process.
Tools designed for scenario-finding produce examples of how an artifact can behave in practice. Scenario-finders often allow the results to be targeted to a desired output or test a particular portion of the artifact—e.g., producing counterexamples that disprove an assumption—and so they are fundamentally different from most forms of testing or simulation. Even better, concrete scenarios appeal to the intuition of the developer, revealing corner-cases and potential bugs that may never have been considered.
Alloy
The Alloy Analyzer is a popular light-weight scenario-finding tool. Let's look at at a small example: a (partial) specification of the Java type system that is included in the Alloy distribution. The explanations below each portion are quoted from comments in the example's source file.
abstract sig Type {subtypes: set Type} sig Class, Interface extends Type {} one sig Object extends Class {} sig Instance {type: Class} sig Variable {holds: lone Instance, type: Type}
fact TypeHierarchy { Type in Object.*subtypes no t: Type | t in t.^subtypes all t: Type | lone t.~subtypes & Class } fact TypeSoundness { all v: Variable | v.holds.type in v.type.*subtypes }
Alloy will let us examine how the model can behave, up to user-provided constraints. We can tell Alloy to show us scenarios that also meet some additional constraints:
run { some Class - Object some Interface some Variable.type & Interface } for 4
Alloy always checks for scenarios up to a finite bound on each top-level type—4 in this case. Here is the one that Alloy gives:
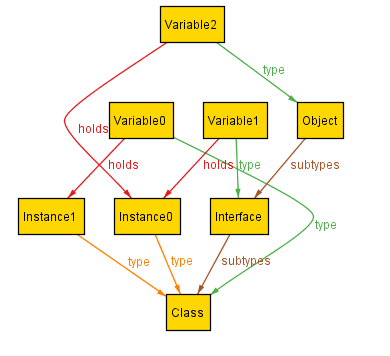
This scenario illustrates a possible instance of the Java type system. It's got one class and one interface definition; the interface extends Object (by default), and the class implements the interface. There are two instances of that class. Variable0 and Variable1 both hold Instance1, and Variable2 holds Instance0.
Alloy provides a Next button that will give us more examples, when they exist. If we keep clicking it, we get a parade of hundreds more:
![]() |
![]() |
![]() |
Even a small specification like this one, with a relatively small size bound (like 4), can yield many hundreds of scenarios. That's after Alloy has automatically ruled out lots of superfluous scenarios that would be isomorphic to those already seen. Scenario-overload means that the pathological examples--the ones that the user needs to see--may be buried beneath many normal ones.
In addition, the order in which scenarios appear is up to the internal SAT-solver that Alloy uses. There is no way for a user to direct which scenario they see next without stopping their exploration, returning to the specification, adding appropriate constraints, and starting the parade of scenarios afresh. What if we could reduce the hundreds of scenarios that Alloy gives down to just a few, each of which represented many other scenarios in a precise way? What if we could let a user's goals guide their exploration without forcing a restart? It turns out that we can.
Aluminum: Beyond the Next Button
We have created a modified version of Alloy that we call Aluminum. Aluminum produces fewer, more concise scenarios and gives users additional control over their exploration. Let's look at Aluminum running on the same example as before, versus the first scenario that Alloy returned:
![]() The 1st Scenario from Alloy |
![]() |
Compared to the scenario that Alloy produced, this one is quite small. That's not all, however: Aluminum guarantees that it is minimal: nothing can be removed from it without violating the specification! Above and beyond just seeing a smaller concrete example, a minimal scenario embodies necessity and gives users a better sense of the dependencies in the specification.
There are usually far fewer minimal scenarios than scenarios overall. If we keep clicking Next, we get:
![]() |
![]() |
Asking for a 4th scenario via Next results in an error. That's because in this case, there are only three minimal scenarios.
What if...?
Alloy's random, rich scenarios may contain useful information that minimal scenarios do not. For instance, the Alloy scenarios we saw allowed multiple variables to exist and classes to be instantiated. None of the minimal scenarios illustrate these possibilities.
Moreover, a torrent of scenarios--minimal or otherwise--doesn't encourage a user to really explore what can happen. After seeing a scenario, a user may ask whether various changes are possible. For instance, "Can I add another variable to this scenario?" More subtle (but just as important) is: "What happens if I add another variable?" Aluminum can answer that question.
To find out, we instruct Aluminum to try augmenting the starting scenario with a new variable. Aluminum produces a fresh series of scenarios, each of which illustrates a way that that the variable can be added:
![]() |
![]() |
![]() |
(The newly added variable is Variable0.)
From these, we learn that the new variable must have a type. In fact, these new scenarios cover the three possible types that the variable can receive.
It's worth mentioning that the three augmented scenarios are actually minimal themselves, just over a more restricted space—the scenarios containing the original one, plus an added variable. This ensures that the consequences shown are all truly necessary.
More Information
To learn more about Aluminum, see our paper and watch our video here or download the tool here.
ADsafety
Tags: Browsers, JavaScript, Programming Languages, Security, Types, Verification
Posted on 13 September 2011.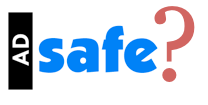
A mashup is a webpage that mixes and mashes content from various sources. Facebook apps, Google gadgets, and various websites with embedded maps are obvious examples of mashups. However, there is an even more pervasive use case of mashups on the Web. Any webpage that displays third-party ads is a mashup. It's well known that third-party content can include third-party cookies; your browser can even block these if you're concerned about "tracking cookies". However, third party content can also include third-party JavaScript that can do all sorts of wonderful and malicious things (just some examples).
Is it possible to safely embed untrusted JavaScript on a page? Google Caja, Microsoft Web Sandbox, and ADsafe are language-based Web sandboxes that try to do so. Language-based sandboxing is a programming language technique that restricts untrusted code using static and runtime checks and rewriting potential dangerous calls to safe, trusted functions.
Sandboxing JavaScript, with all its corner cases, is particularly hard. A
single bug can easily break the entire sandboxing system. JavaScript sandboxes
do not clearly state their intended guarantees, nor do they clearly argue why
they are safe.
Verifying Web Sandboxes
A year ago, we embarked on a project to verify ADsafe, Douglas Crockford's Web sandbox. ADsafe is admittedly the simplest of the aforementioned sandboxes. But, we were also after the shrimp bounty that Doug offers for sandbox-breaking bugs:
Write a program [...] that calls the alert function when run on any browser. If the program produces no errors when linted with the ADsafe option, then I will buy you a plate of shrimp. (link)A year later, we've produced a USENIX Security paper on our work, which we presented in San Francisco in August. The paper discusses the many common techniques employed by Web sandboxes and discusses the intricacies of their implementations. (TLDR: JavaScript and the DOM are really hard.) Focusing on ADsafe, it precisely states what ADsafety actually means. The meat of the paper is our approach to verifying ADsafe using types. Our verification leverages our earlier work on semantics and types for JavaScript, and also introduces some new techniques:
- Check out the ★s and ☠s in our object types; we use them to type-check "scripty" features of JavaScript. ☠ marks a field as "banned" and ★ specifies the type of all other fields.
- We also characterize JSLint as a type-checker. The Widget type presented in the paper specifies, in 20 lines, the syntactic restrictions of JSLint's ADsafety checks.
Unlike conventional type systems, ours does not prevent runtime errors. After all, stuck programs are safe because they trivially don't execute any code. If you think type systems only catch "method not found" errors, you should have a look at ours.
We found bugs in both ADsafe and JSLint that manifested as type errors. We reported all of them and they were promptly fixed by Doug Crockford. A big thank you to Doug for his encouragement, for answering our many questions, and for buying us every type of shrimp dish in the house.
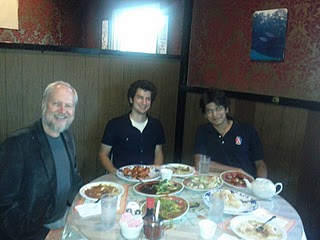
Learn more about ADsafety! Check out:
- The paper, code, and proofs;
- Video of Arjun presenting at USENIX Security;
- ADsafe and JSLint.